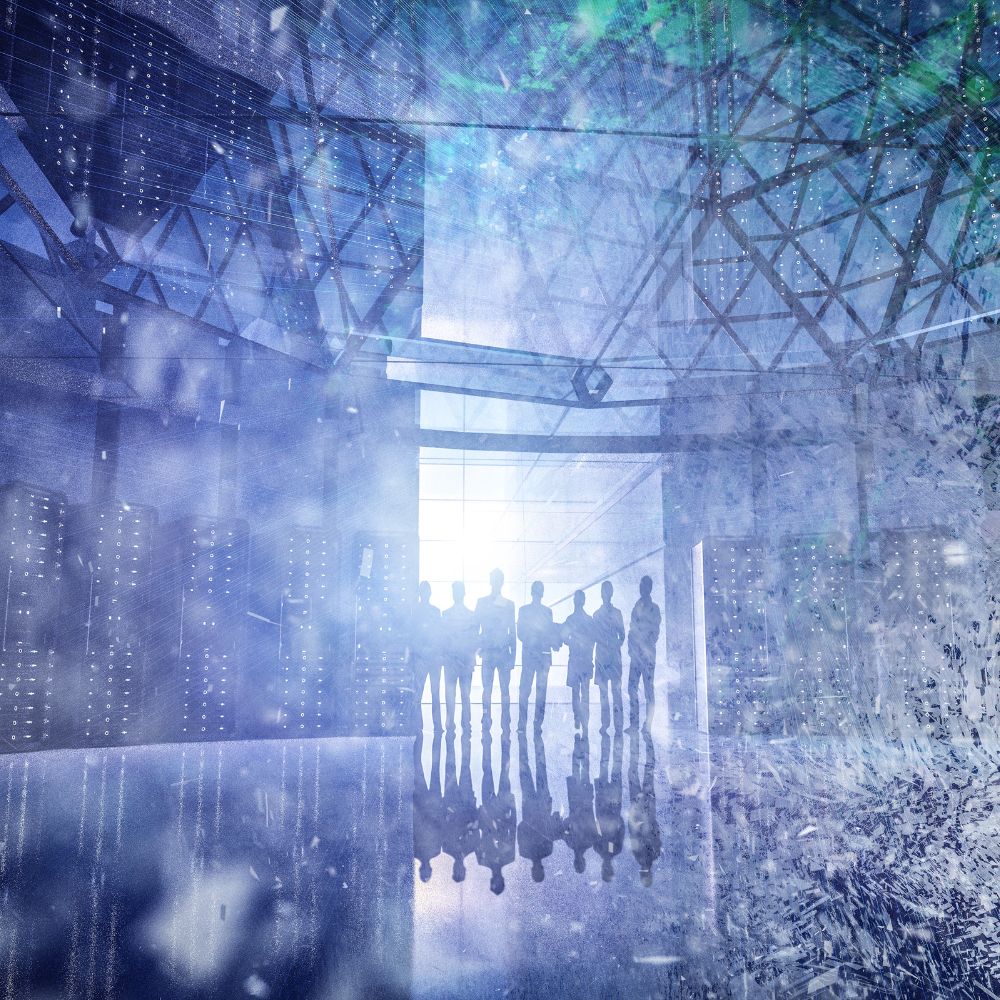
In recent years, there have been incredible strides in the fields of artificial intelligence (AI) and machine learning (ML). These advancements are reshaping various industries, particularly in advertising and marketing. One significant application of AI is in data analysis.
With businesses generating massive amounts of data, AI data analysis emerges as a solution to make sense of this data overload. It transforms raw data into valuable insights, aiding marketers in understanding their target customers. In the past, sifting through such vast data was a challenge, but now, thanks to AI’s prowess, businesses can efficiently filter potential leads and focus their efforts wisely.
Simplify the world of big data with AI
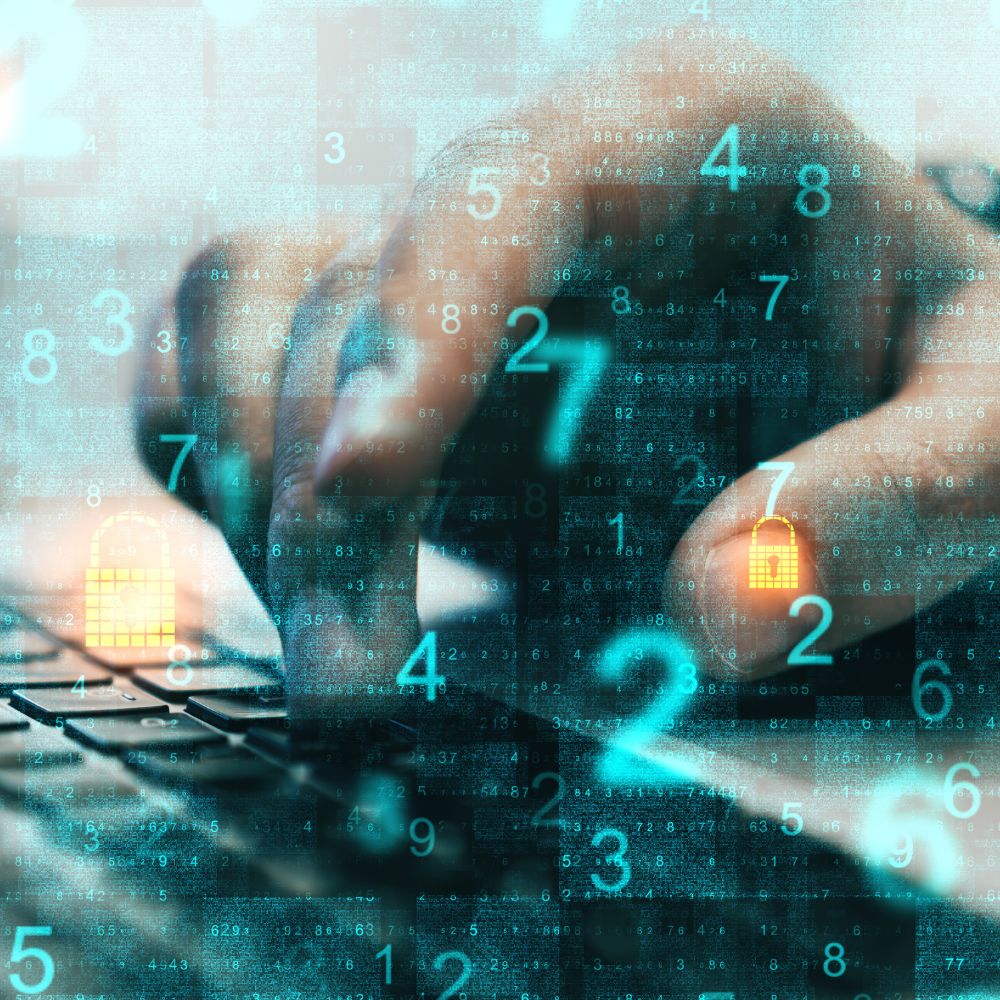
A clear example of AI’s impact can be seen in Google Ads. Have you noticed how advertisements for products you’re interested in seem to follow you online? This is due to AI and ML, which help Google understand user preferences based on online behavior and searches. This way, the ads displayed align closely with individual interests.
The Role of AI in Big Data Analysis: In the realm of technology, “Big Data” refers to data that is complex and extensive, going beyond traditional data analysis methods. AI steps in when conventional approaches fall short. It’s not just about the quantity of data; it’s about transforming it into meaningful information.
Traditional data processing techniques struggle with the massive influx of data types, such as unstructured content like emails, audio, video, and images. Even the Internet of Things contributes to this data surge.
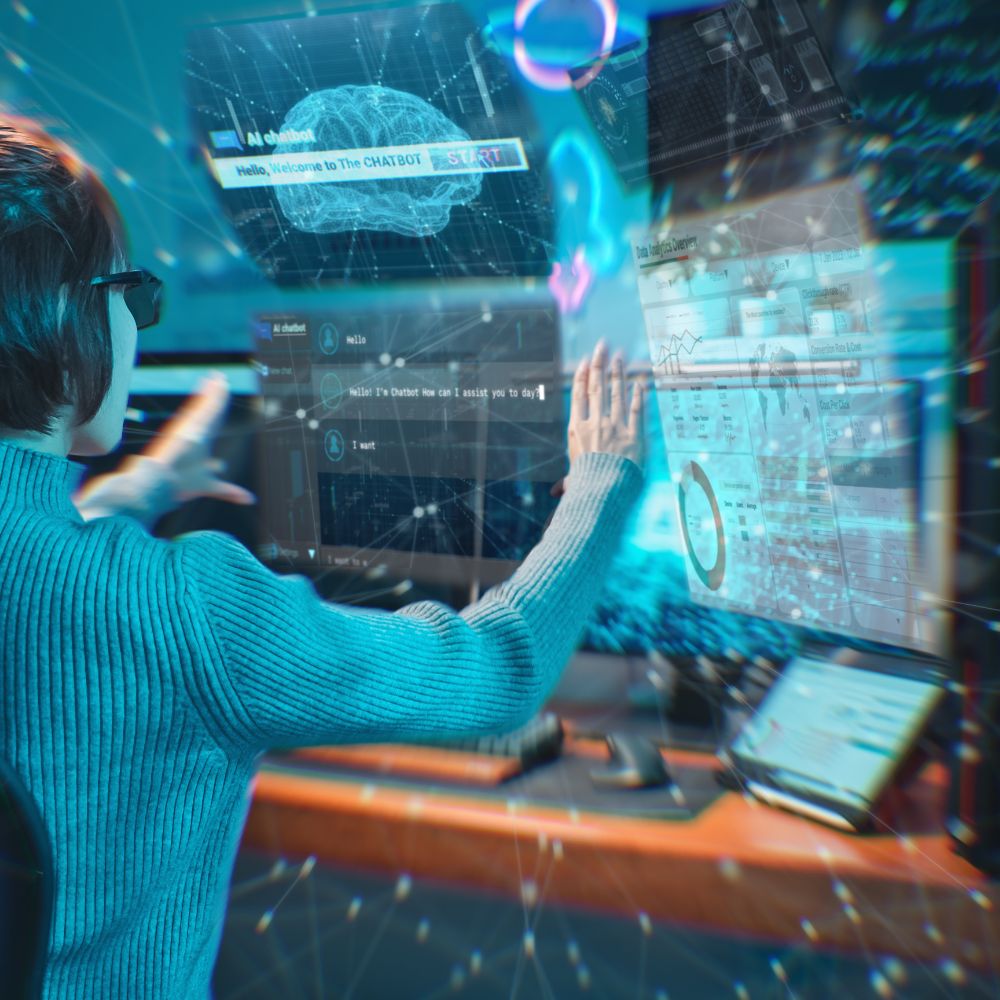
Big Data is often described through the lens of the 3 Vs:
- Volume: the sheer amount of data from various sources
- Velocity is how fast data is created.
- Variety is the mix of data types, including structured, semi-structured, and unstructured data.
To tackle Big Data, AI-driven systems must account for these 3Vs, adjusting their capabilities accordingly.
Understanding Data Analysis: Data analysis involves deciphering patterns within data, encompassing both descriptive analysis (depicting ongoing events) and predictive analysis (forecasting future outcomes based on historical data).
Many businesses grapple with accessing their data efficiently. Often, relevant data exists but lacks tools for analysis. The data might be scattered, requiring consolidation before analysis can even begin. This process sometimes involves comparing different file formats.
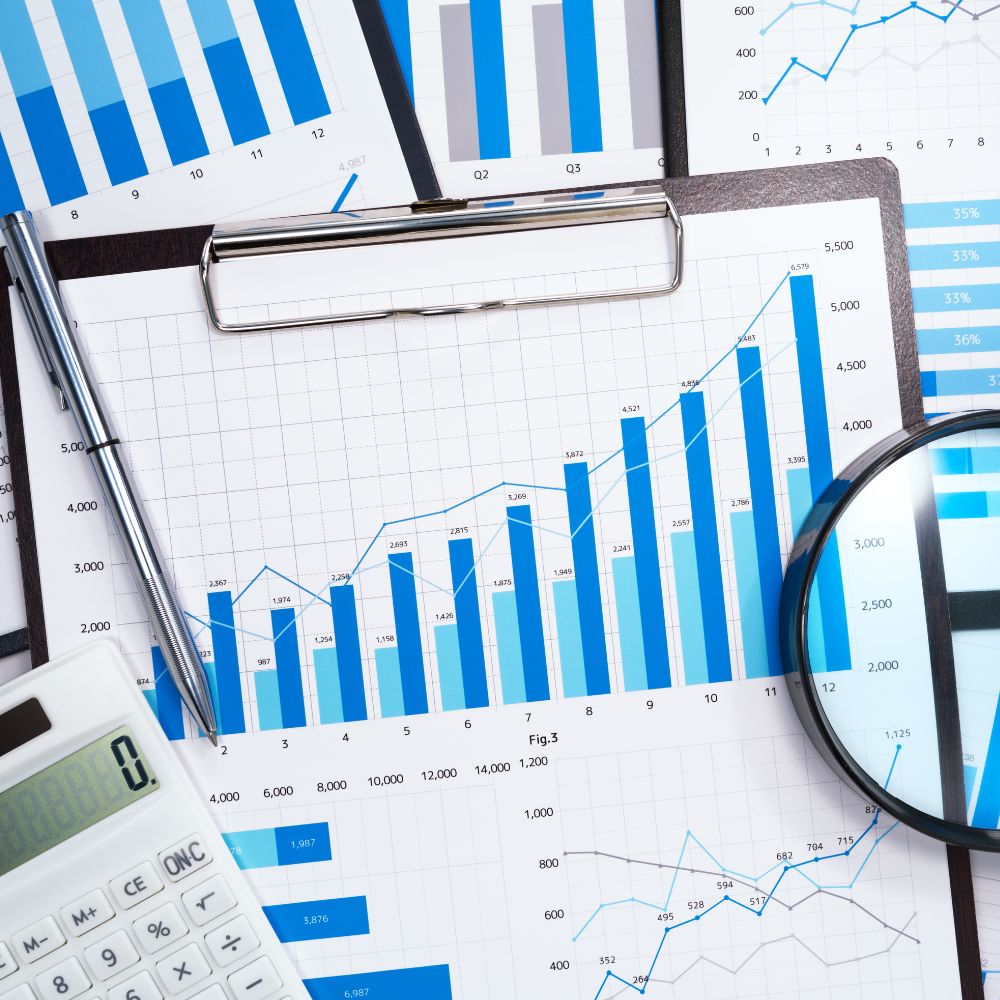
Four main types of data analytics exist:
- Descriptive: answering basic questions about what happened.
- Diagnostic: exploring cause-and-effect relationships
- Predictive: identifying trends and correlations
- Prescriptive: utilizing AI to predict outcomes and recommend actions
Each type serves a specific purpose, aiming to enhance efficiency and performance through data-driven insights.
AI’s Role in Data Analysis

AI’s emergence has transformed the marketing landscape, allowing for faster and more accurate data analysis. AI handles vast datasets, automates complex tasks, customizes content, and predicts outcomes with unprecedented accuracy. It simulates human intelligence processes, learns from training data, and identifies correlations and patterns within live data.
AI utilizes various technologies to tackle Big Data challenges, including predictive analytics, machine learning, and data management. What sets AI apart is its capacity to handle larger volumes of data and work independently, producing superior results.
Unveiling Insights with AI: AI facilitates the discovery of trends and patterns hidden within massive datasets, enabling businesses to optimize systems and offer personalized services.

From analyzing unstructured data like reviews and social media posts to predicting customer needs, AI transforms these insights into actionable strategies. Walmart, for instance, leverages AI to predict inventory needs based on weather data, enhancing customer satisfaction.
AI’s potential for uncovering customer insights is vast. Text analytics reveals sentiment, trends, and discussion topics. Predictive and prescriptive analytics empower businesses to anticipate customer desires, thereby improving efficiency and customer value.
Here are the top 3 AI tools for 2023 to help you analyze data effectively

Microsoft Power BI
Microsoft Power BI is a robust data analysis tool that helps users visualize and analyze data from various sources. It has an easy-to-use interface, allowing organizations to turn complex data into meaningful insights. With interactive dashboards, data exploration, and advanced analytics, Power BI is favored for its integration with Microsoft products, making it a top choice for businesses.
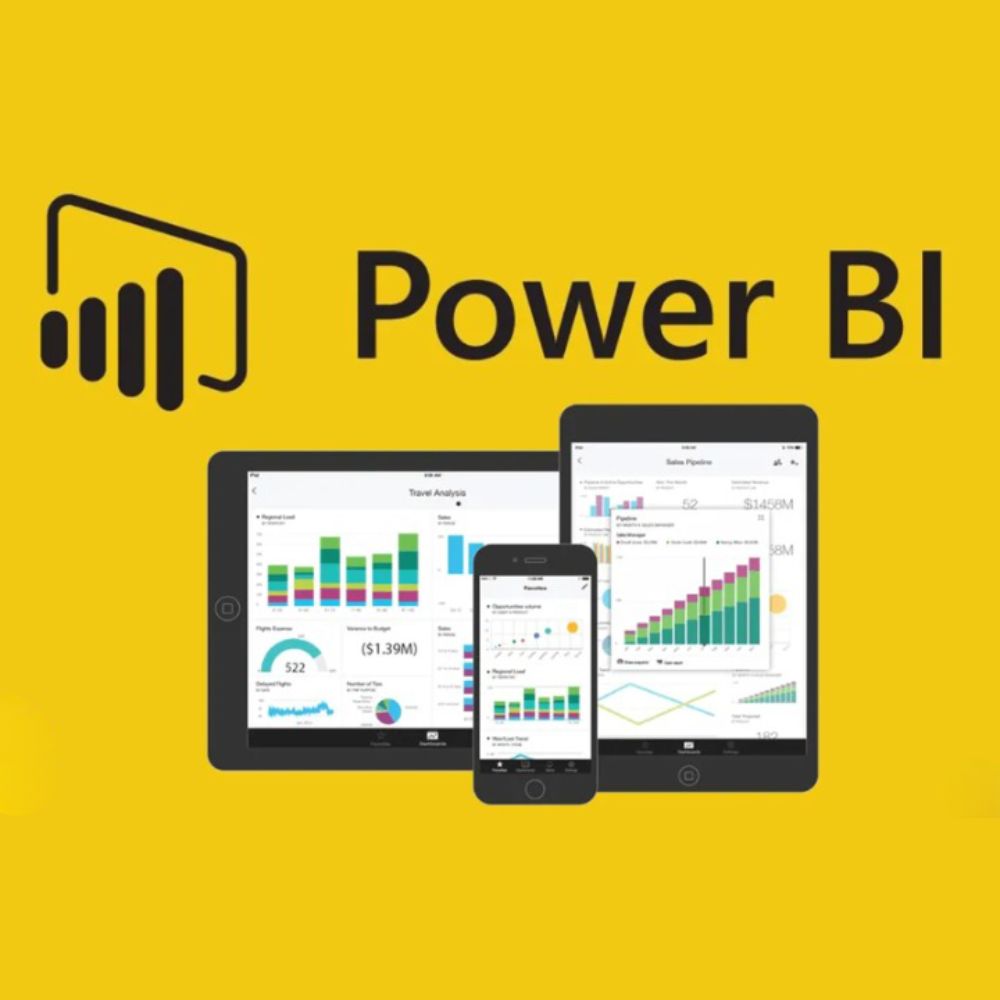
Advanced analytics features enable statistical analysis, data modeling, and predictive modeling with machine learning, aiding data-driven decisions. Sharing features ensure up-to-date reports and dashboards can be securely accessed by teams.
Tableau

Tableau is another leading data analysis tool known for its intuitive interface and advanced capabilities. It supports various data sources and lets users create interactive dashboards and reports effortlessly. Advanced analytics include statistical modeling, forecasting, and the integration of predictive analytics models. Collaboration is simplified through sharing visualizations and dashboards, facilitating real-time teamwork.
Qlik Sense
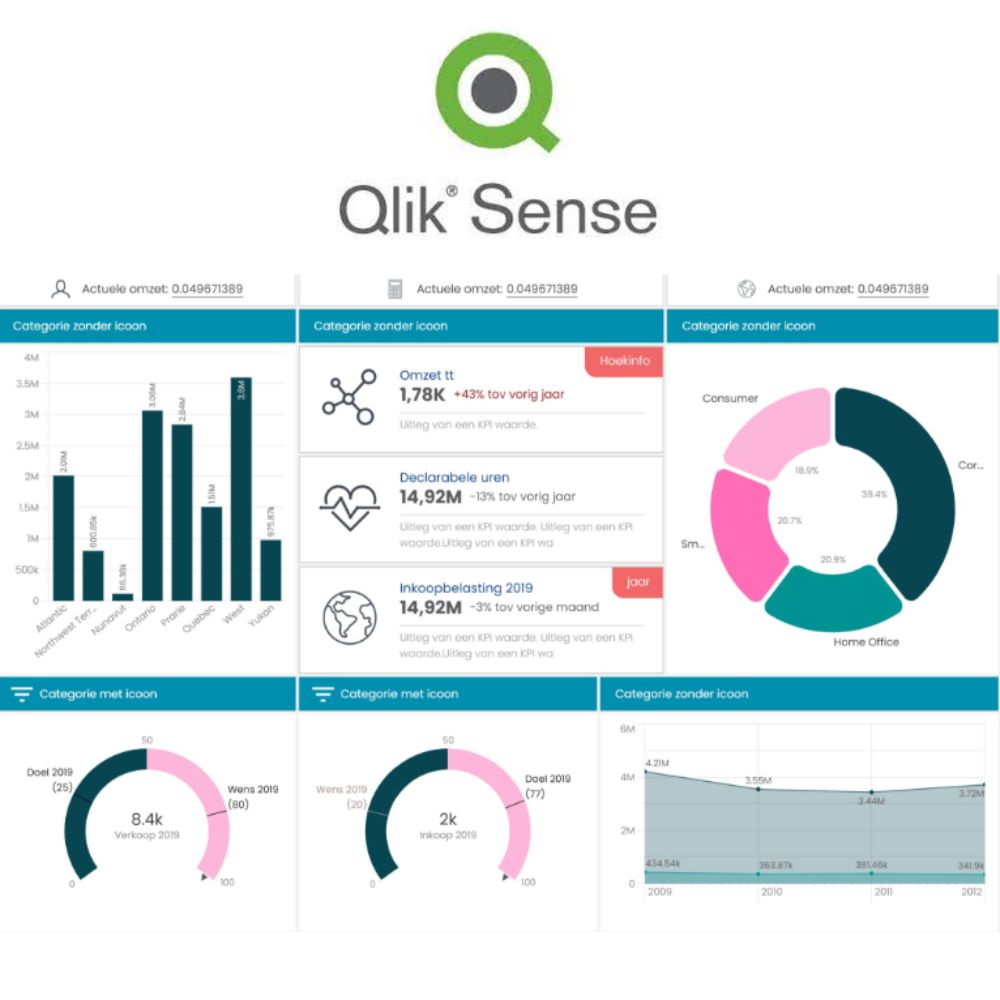
Qlik Sense is a user-friendly data analysis and visualization tool that empowers users to explore data efficiently. Its associative data model links diverse data sources for a comprehensive view. Qlik Sense’s strength lies in its self-service approach, allowing users to create visualizations and discover insights without coding. The interactive interface aids in navigating data, revealing patterns and insights easily.
Article conclusion
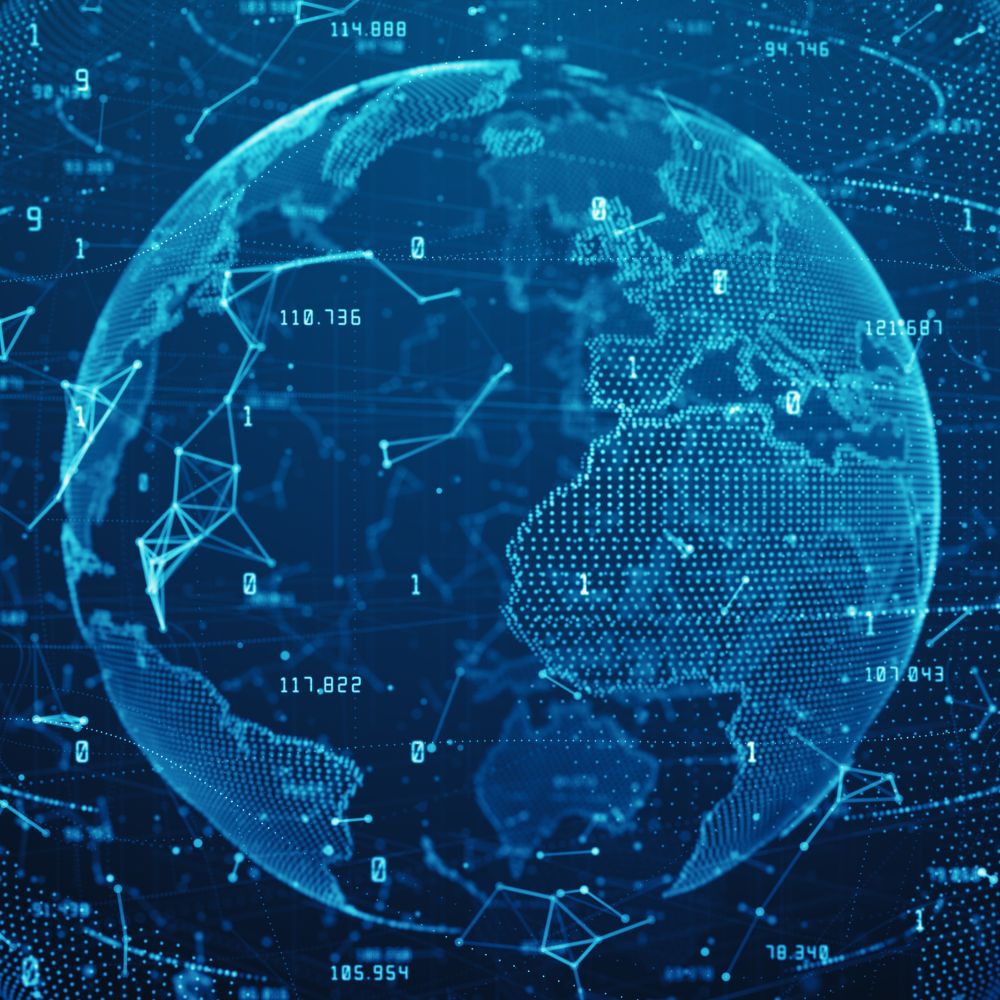
In a nutshell, AI revolutionizes data analysis by automating labor-intensive tasks, handling vast datasets, and offering objective insights that drive better business decisions. Its influence is transforming how businesses glean insights from their data, elevating operations and customer experiences alike.
Related posts: Analyzing Data (2023): YouTube Analytics Fundamentals
Discussion about this post